Experimental characterisation of construction materials
Experimental characterisation of materials is needed to formulate appropriate computational modelling strategies and enables the development of new materials with improved properties. We are particularly interested in the inverse identification of constitutive parameters using full-field imaging data and categorising and localising internal failures using acoustic emission data. Our current applications in this area relate to characterising elasticity and fracture in lime mortar and rocks, failure modes in fibrous plaster, creep and interface properties in masonry, and shape memory effects in iron-based alloys. We are also interested in exploring the links between physical, chemical and mechanical characteristics of the investigated materials at micro and macro scales. As a part of this effort, we are investigating the impact of microstructure changes due to processes involving hydration, carbonation, corrosion, salt attack and freeze-thaw cycles.
Research themes: Masonry, Historical construction, Experimental mechanics, Structural health monitoring, Computational mechanics.
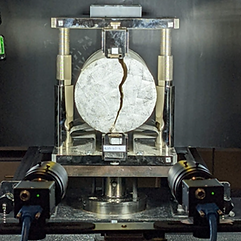
Computational modelling of damage and degradation in construction materials
We are interested in the development of physics-based computational models to describe the damage and degradation processes in construction materials. These simulations often require coupling multiple physical phenomena and operate across scales. For the past few years, we have been developing coupled soil-structure interaction models to predict damage due to underground construction induced ground movements. In our ongoing projects, we are investigating simulating fracture in quasi-brittle materials, damage in buildings due to climate change induced soil moisture variations and automated generation of damaged masonry arch bridge point clouds from computational models.
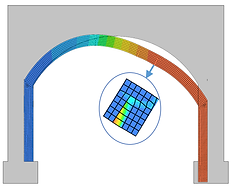
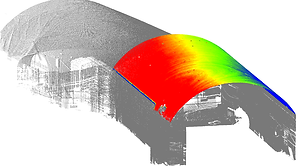
3D model analysis for structural assessments
3D models can be generated using laser scanning or photogrammetry and are commonly used to document large civil engineering structures. We are developing new segmentation, registration and point cloud comparison algorithms to derive new insight from this data. These inform various applications in defect detection, full-field displacement monitoring (by using consecutive point clouds) and reconstruction of historical displacements (from assumed initial geometries and current 3D geometry). The use of deep learning to improve and automate these techniques is a new research focus.